We are witnessing advanced & dramatic growth in Business Intelligence and how individuals & businesses are taking advantage of it. Though for that to happen it is essential to be able to analyze and utilize data. And, for that, all the data needs to be stored in one place & structured to harness & analyze it.
And, implementing an Enterprise Data Warehouse (EDW) can be a great way to support your digital transformation journey. A data warehouse stores large data sets from multiple data sources to help derive valuable insights for informed decision-making. But building a warehouse is not simple.
Through this article, we will take a deep dive into the details of why & how to build a data warehouse. So, if you are looking to get outlines on the fundamental approach to EDW design & development, this post will be worth reading.
Here’s what we will cover.
Why Does Your Business Need a Data Warehouse?
Having your business data gathered in one place is one of the biggest advantages of having a DWH. Though it is not the only one. Data warehouse development enables you to leverage additional benefits such as:
- Saving time on data retrieval from multiple sources
- Elimination of manual errors in data aggregation
- Easy availability of structured data
- Automation of data management processes like collection, structuring, etc.
- Supportive platform for advanced data analytics
- Better data security due to a unified approach
Core Components of Data Warehouse Architecture
Listed below are critical components you need to consider while developing your future data warehouse. It would include how many data sources will connect to DWH, the amount of information segregated by their nature & complexity, analytics objectives & more.
A typical data warehouse architecture has the following layers:
Data source layer
- Collects data from all your data sources (both internal & external)
- Makes the data readily available for processing in the staging layer
- Varied data sources have different business & data processing cycles, geographical variables, network & hardware resource restrictions, etc. So, it is impossible to collect data from all the sources at once
- Examples of source data include data from web browsers, IoT devices, social media, internal applications, other databases, etc
Data staging layer
- Extracts data from the source layer and stores it in the temporary landing database with the Extract, Transform, and Load (ETL) process
- Identification of schema & structure, cleansing, formatting, structuring, testing, etc.
- Depending on the methodologies, this layer may not be needed in certain instances if the storage layer carries out the ETL process.
Data storage layer
- Hosts the data warehouse database for company-wide information.
- Enables hosting data marts that are the subsets of your data warehouse & store business area-specific data.
- Ensures data availability for end users data is available for end users.
Note: Data analytics & Business Intelligence tools are further integrated on the top of the data storage layer.
Data Warehouse Development Approaches
Your data warehouse must adapt to the requirements of your business users from varied functional areas like HR, Supply Chain, Finance, etc. We understand that every data warehouse is unique and requires a different approach to development. The traditional approaches used to create a data warehouse include top-down & bottom-up.
Top-down Approach
Referred to as Bill Inmon’s approach, it deals with designing the centralized storage first and then the creation of data marts from summarized DWH data.
- Follows relation modeling
- Is IT-driven where users have a passive participation
- 3-tier (involves data warehouse, data mart, cube)
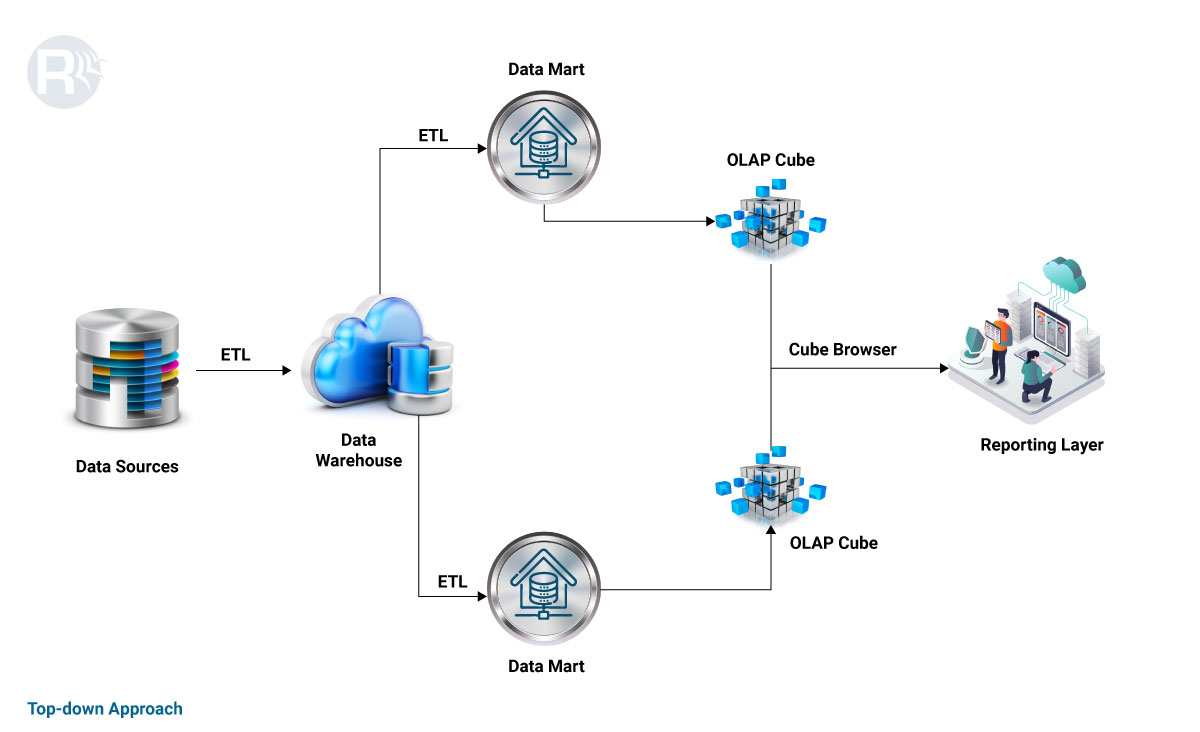
Pros
- Integrated & flexible architecture that supports analytic data structures like data warehouses & data marts
- Consistent & standardized data across data marts offering a single version of the truth
Cons
- Takes longer and is costlier to deploy, especially with initial increments
- The reports are extracted from data marts. And the underlying summary data is in the data warehouse. Therefore, it is difficult for users to locate the summary data by finding a connection between the data mart & the data warehouse.
Bottom-up Approach
Referred to as Ralph Kimball’s approach it suggests building data marts first followed by incremental development of the data warehouse from independent data marts.
- Follows dimensional modeling
- Is business-driven with active participation from the users
- Relies on a “dimensional bus” to logically integrate data marts
- 2-tier (involves data mart, cube)
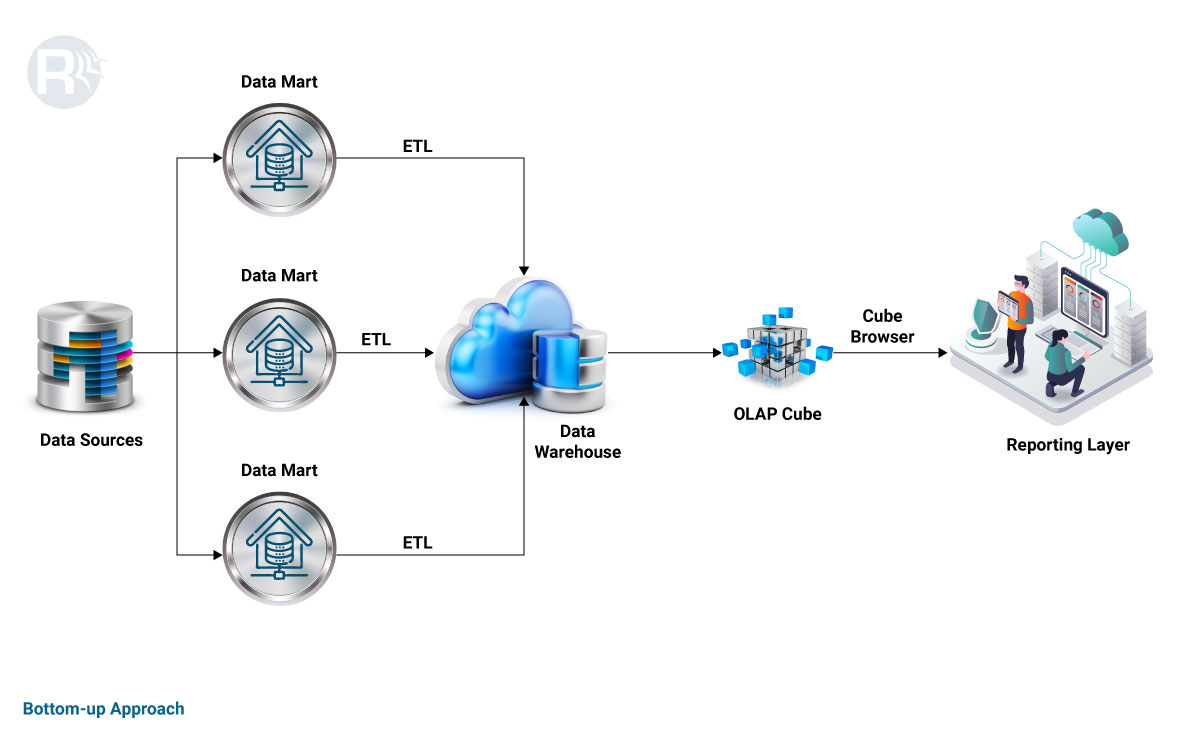
Pros
- Creates flexible data structures to allow analytics & reporting for different business areas
- No up-front heavy investment in infrastructure
- Offers rapid business visibility
Cons
As different business areas may not follow the same rules & references for data management, the data marts
- Could be non-integrated
- May lack data consistency
- Possibility of redundant data
While it is not always feasible to rely on any one approach because both approaches have their pros & cons.
As an experienced data warehouse consulting and development services company, we customize the approaches to meet business needs. And follow a hybrid or federated approach, wherever applicable.
Hybrid Approach
Combines the best of both approaches while utilizing;
- Data integration capabilities of the top-down approach
- Speed & user orientation of the bottom-up approach
This approach emphasizes developing a normal enterprise model and the first dependent data mart. Enables designing first several independent data marts in the normal form while using the star schema physical models to deploy them. Further, an ETL tool stores & manages the dependent & independent mart models. This is while synchronizing the variances in data. It also extracts & loads data from source systems into the independent data marts at atomic & summary levels.
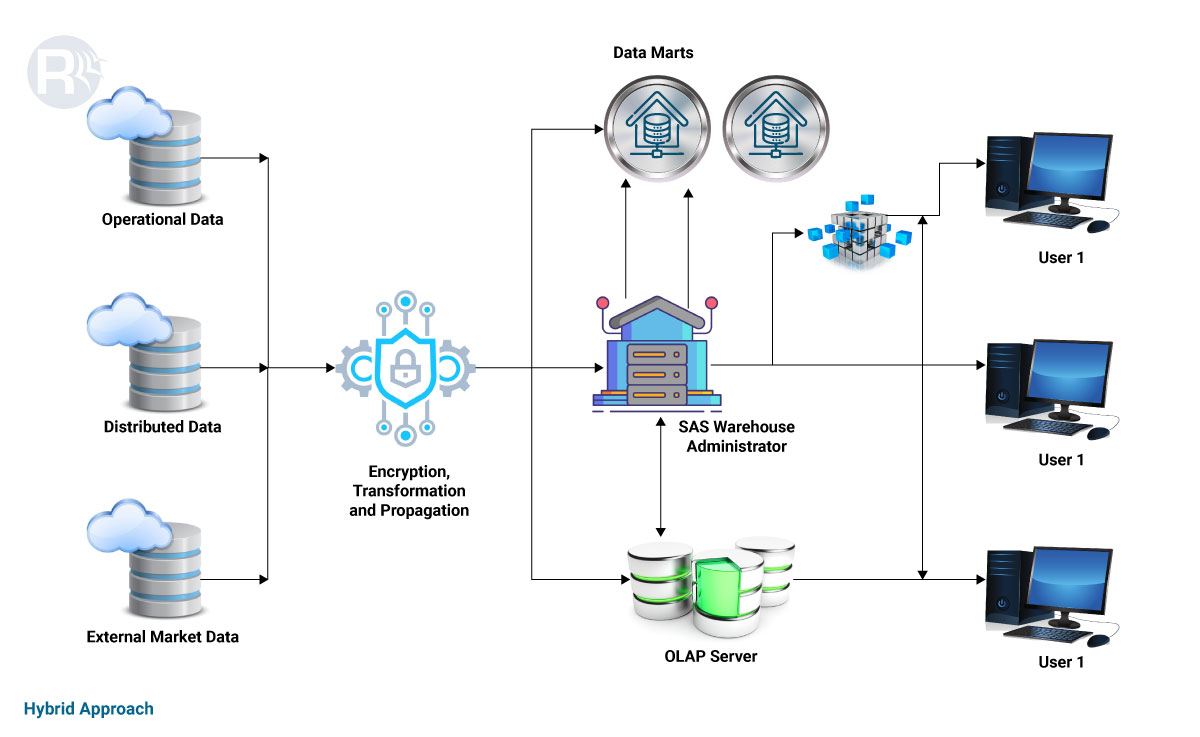
Pros:
- Rapid development
- Enterprise architecture framework
- Possibility to query both atomic & summary data
Cons:
- Heavy reliance on an ETL tool
- Limited choice of querying tools available
Federated Approach
It rationalizes using any means possible to integrate analytical resources & meet changing business needs. This approach acknowledges the reality that organizations & systems change over time to implement a formalized architecture. It emphasizes;
- Integrating new and existing heterogeneous packaged applications, data warehouses, data marts, BI environments, etc
- Creation of a common staging area for organizational level – metrics, data, dimensions, definitions, facts, rules, etc. This would help eliminate redundant data feeds. This would be about creating a data warehouse from multiple analytic applications, data marts, or data warehouses
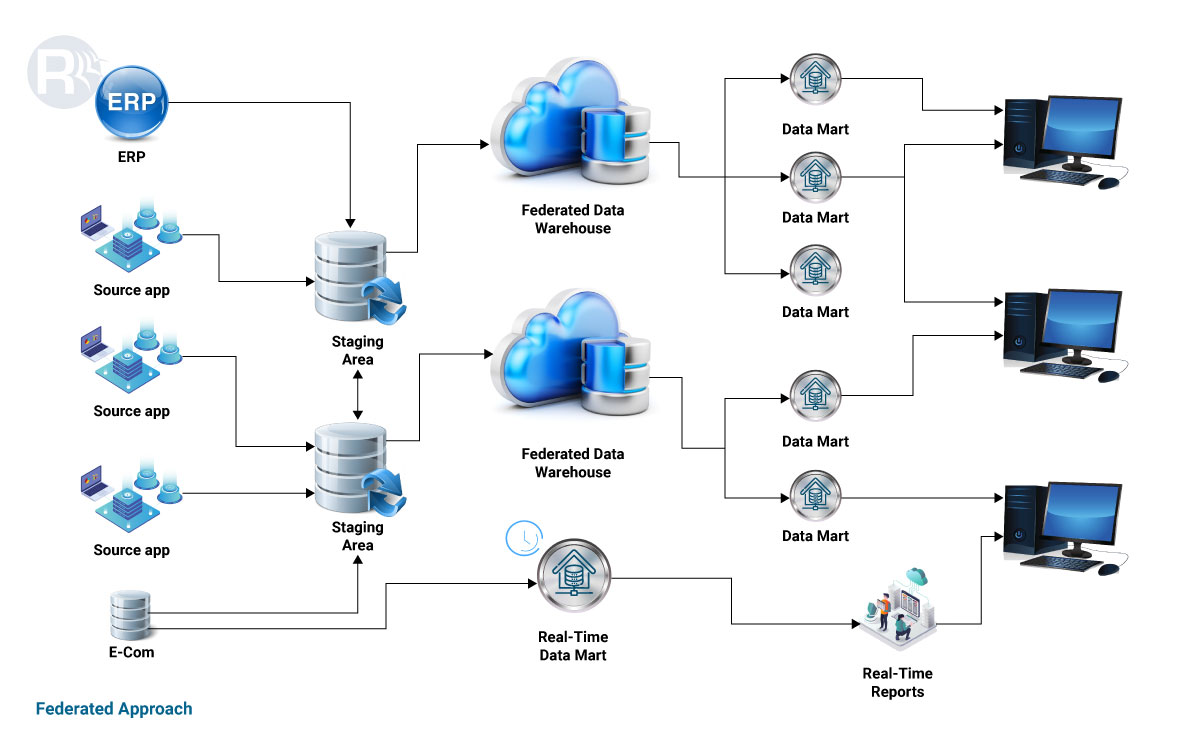
Pros:
- Solves data management issues arising due to organizational changes
- Does not require adhering to formalized architectures
- Allow sensible sharing of data & resources
Cons:
- Not a well-documented approach
- No predefined end-state or architecture
- Might encourage independent development & disintegrate data standardization
DWH Industry Use Cases
Fintech
Customer Data Management
Financial firms may collect every client interaction with data warehouses. It allows them to;
- Examine what influences purchasing decisions and other consumer behaviors
- By quickly recording and tracking large quantities of consumer & historical data from various sources
Insight Management
DWH plays a significant role in offering you predictive & real-time analytics. They help with;
- Storing data centrally about critical financial details to access historical information quickly
- Discover patterns based on customer insights and fraud detection input
Risk Management
By utilizing machine learning algorithms, DWH helps automate the process of risk management. It allows;
- Streamlining data analysis and managing risks
- Centralize data from multiple sources to speed up analytics from multiple user roles
Travel & Hospitality Industry
Booking and property data consolidation
To work as a single source of truth for the following data points;
- Business unit, department-wise reports & dashboards
- Helps track overall company performance
- Distribution channel performance – for distribution channels website, online travel agencies, online booking platforms, metasearch platforms & more)
- Key performance metrics (KPIs) assessment for departments, processes & employees across the board
Housekeeping data
To identify gaps & discover opportunities for cost-reduction & quality improvement across.
- Working staff count in real-time
- Cleaning timelines for stay-over vs checkout
- Performance indicators (number of rooms per chambermaid; rating for the quality of cleaning)
- Inventory related – linen availability, cleaning supplies & chemicals & amenities cost
- Laundry expenses
- Repair information
- Addressal of guest requests & complaints
Room Tariff Information
It is vital to set a pricing strategy by implementing a rate shopping software (for rooms & travel) integrated with a data warehouse.
- This would include direct API connections to map competition based on their sales revenues
- Visibility provides the opportunity to identify performance gaps
Guest data
The CRM platforms integrated with DWH allow the creation, access & analysis of guest profiles while obtaining a true “picture” of each customer. The list of valuable attributes recorded in the said profile would include;
- Contact information — phone number, physical and e-mail address;
- Demographics — age, marital status, number of children;
- Reservation data & booking channel preferences with past booking history
- Reasons for stay and services utilized with feedback on each
- Food and beverage (F&B) preferences
- Cleaning notes and housekeeping preferences;
- Revenue persona
- Payment mode
- Loyalty level/status
Retail Industry
Demand Forecasting
An essential feature for retailers that enables them to scale operations
- Predict & meet the increased demand during peak seasons
- Scale down to avoid excess inventory during the normal sales cycle
- Business unit, department-wise reports & dashboards
In-depth Customer Understanding
DWH assists retailers with getting a deeper look at customer & their interests
- Helps store, process & analyze customer’s buying behavior and preferences
- Utilizing the insights for customized offers, contextual marketing & even redesigning the aesthetics of both online & physical stores
Enhanced Business Intelligence
Enables performance tracking available across department, process & employee levels
- Self-service capabilities of the dashboard to perform drill-down and drill-up functions to view the data at a holistic as well as granular level
- Inventory management, supplier integration, shelf space allocations
- Business operation consolidation & analytics led prediction
- Performance assessment and optimization
- Business forecasting, sales analysis, target marketing
- Tracking the contribution of each product within its category
Steps to Build a Data Warehouse
Based on our experience in DWH development, here’s a suggestive plan to create a data warehouse from scratch. However, some steps may vary depending on project complexity, data quality, data analytics objectives & more.
Information Gathering
- Understanding your short & long-term business needs & expectations
- Listing all your business areas & recognizing the requirements of end-users in each area
- A thorough assessment of your existing IT infrastructure including software, architecture, third-party integrations, etc.
- Initial analyzing your data sources for quality, volume, structure, type, etc.
- Summarizing the data warehouse solution requirements including possible integrations, etc.
- Identification of the development team needed for this project including talents like project manager, business analyst, system analyst, solution architect, data engineer, quality assurance engineer, and DevOps engineer.
Ideation
- Defining the desired features to be built for your data warehouse
- Selecting the most suitable approach for architecture
- Determining the deployment option that best suits your business like on-premise, cloud, or hybrid
- Deciding the platform and DWH technologies for your project including database type, data modeling tools, ETL tools, analytics tools, BI tools, etc.
Project Roadmap
- Creating the project scope, timelines, budget planning & more
- Planning for the data warehouse design and development
- Crafting the DWH development scope by including solution architecture vision document, data warehouse deployment strategy, testing strategy & project implementation
- Essential risk management plan considerations
- Effort estimation for the DWH project, TCO & achievable ROI
Architecture Design
- Detailed analysis of all your data sources including;
- Identifying the controls & limitations for data access
- Knowing the type, structure, volume & quality of data
- Understanding the existing data flows between data sources
- Detailing out the data update schedules for each data source
- Creating policies for data security & cleansing
- Designing data models for both the data warehouse & the data marts
- Discovering data objects & the relationship between them
- Incorporating data objects into the data warehouse
- Designing ETL/ELT processes for data integration and data flow control
- Defining data flow & integration controls & processes
Development & Testing
- Developing ETL/ELT data pipelines, data lakes, etc.
- Perform ETL/ELT Testing
- Implementation of security controls at the data level
- Customization of the data warehouse platform
- Testing of all the data flow & management processes
- Conducting overall performance testing of the data warehouse
Deployment
- Data migration
- Assessment of data quality
- Providing access to business users
- Conducting training for users
- Assessing user acceptance
Post-deployment Support
- Providing support for the smooth running of the data warehouse solution
- Enhancing the DWH performance and availability as needed
- Performance-tuning of ETL/ELT processes
Data Warehouse Software/Tools
We utilize reliable platforms from industry leaders like AWS and Azure to build high-performing data warehouses.
Some of them include:
Azure Synapse Analytics
- Offers 95+ native connectors for cloud data & on-premises sources via Azure Data Factory
- Built-in Apache Spark and Azure Stream Analytics event-processing engine to support big data, streaming data ingestion & processing
- Allows native integrations with Power BI, Azure Stream Analytics, Apache Spark, Azure Cognitive Services, Azure Cosmos DB, Azure ML, etc.
- Supports multiple languages like .Net, Python, T-SQL, Spark SQL, Scala
- Native HTAP support via Azure Synapse Link
- Supports disaster recovery capabilities with built-in fault tolerance
- A separate process for scaling storage and computation
- Helps meet compliance requirements for HIPAA, ISO 27001, PCI DSS, SOC1, SOC2 and more
Explore how we utilize Azure Analytics Services to help organizations leverage cloud-based analytics.
Amazon Redshift
- AWS ecosystem integration with Amazon S3, Amazon Athena, Amazon SageMaker, Amazon EMR and more
- Offers data ingestion and transformation (in both stream/batch and within/outside of AWS services) with AWS Data Pipeline, AWS Data Migration Services, AWS Glue and AWS Kinesis Firehose
- Integration with third-party tools like Cloud, Power BI, data modeling tools, etc.
- Allows live querying of data across databases like RDS MySQL, Amazon Relational Database Service (RDS), Aurora MySQL, and Aurora PostgreSQL
- A separate process for scaling storage and computation
- Helps meet compliance requirements for SOC1, SOC2, SOC3, PCI DSS Level 1, HIPAA, ISO 27001
Cost Considerations for Building a Data Warehouse
While all data warehouses are unique in their own way it becomes difficult to attribute a fixed cost to build one. Though typically for data warehouse development listed below are the factors that influence the cost:
- The number & different types of data sources like web & mobile apps, social media, IoT devices, etc.
- The difference between the structures & formats of data sources
- Data Volume, Complexity & Sensitivity
- Data security needs
- The number of data flows & data objects required
- The speed, scalability & other performance requirements of the data warehouse
Concluding Thoughts
We hope this guide clears all your questions & doubts about how to build a data warehouse. At Rishabh Software, we have the experience & expertise to provide custom DWH development to meet your business & technology needs.